How We Select Managers for EAM
About Pegassets
The principals of Pegassets have extensive investment industry experience and have been designing EAM strategies since 2019. Both Robert Tull and Kathleen Neumann, CFA are members of Turing Technology’s Board of Advisors.
Bob Tull is a well-recognized and award-winning expert in the ETF market since 1993. He has played a leading role in the design and development of over 400 exchange traded products (e.g., ETFs) in the U.S., Europe and Pacific Rim, and holds multiple patents related to exchange traded products.
Kathy Neumann brings more than 30 years' experience in the investment management industry including president of TAMRO Capital Partners, a $2.5 billion asset management firm, as well as president of SerenityShares Investments, an ESG-dedicated ETF start-up. Kathy holds the Chartered Financial Analyst designation and is a past President of the CFA Society of Washington.
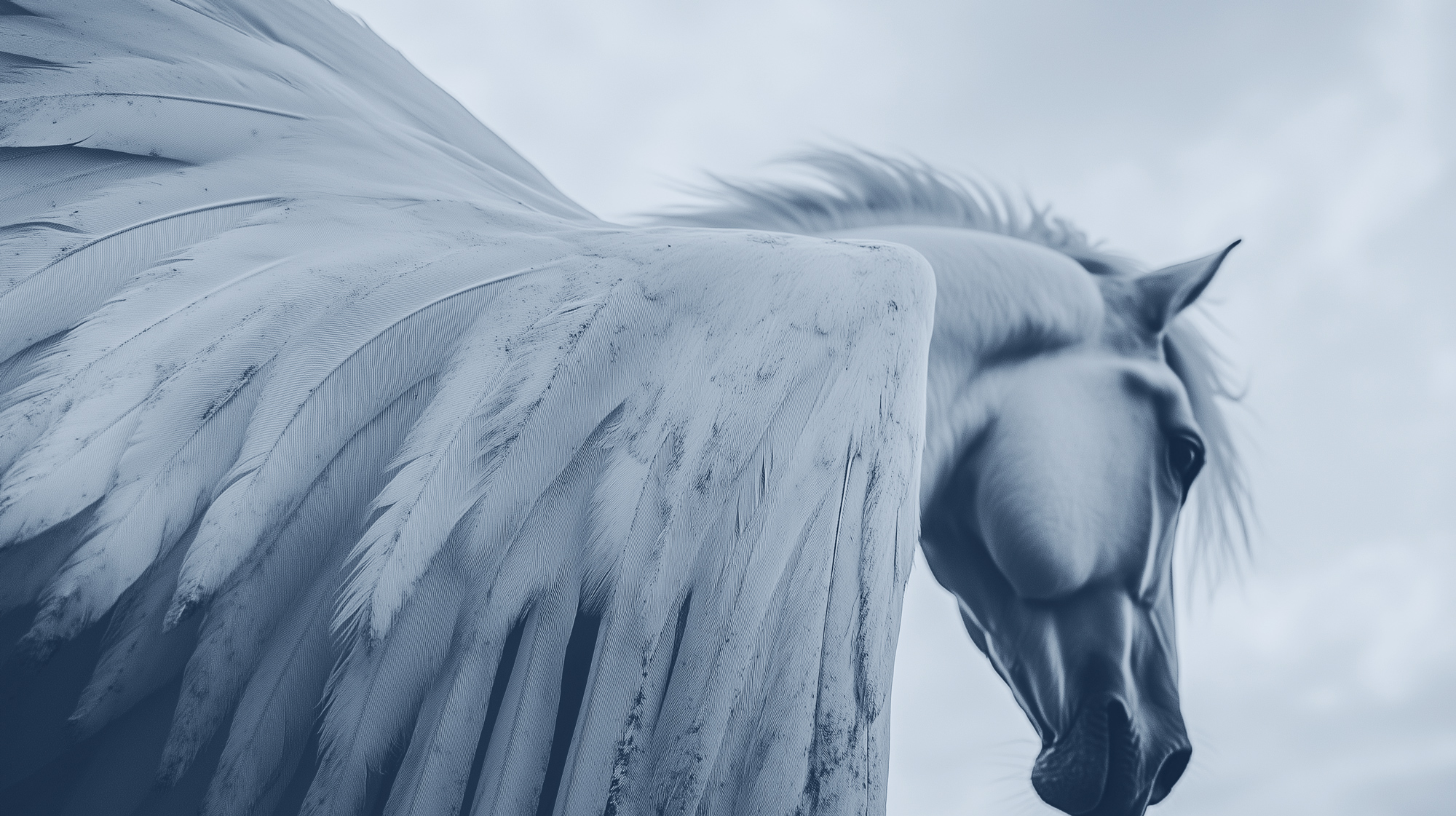
Core Philosophy
Pegassets’ Underlying Fund selection process has been refined over the years based on lessons learned as to what characteristics lead to successful outcomes. This includes insights into the optimal time periods to measure manager “skill” (longer is better), the importance of style consistency (very), and how to embed defensive biases into the manager selection process (defensive bias is a targeted outcome of most of our strategies).
Our fund selection process is also informed by a deep and experienced understanding of how the Ensemble Active Management technology translates manager stock decisions from Underlying Funds to the generation of “Ensemble AlphaTM,” the key driver of EAM portfolio outperformance.
The desired performance attributes of Pegassets’ EAM strategies that we target include the following:
- Persistent ability to outperform the benchmark
- Quality risk-adjusted performance
- A defensive bias
- Capacity to outperform in up markets as well as negative markets
To achieve this goal, we focus on several key elements in our selection process for the Underlying Funds that form the foundation of Pegassets’ design framework (not in order of priority):
- Independence of investment process and approach (e.g., never more than one fund from the same fund family)
- Demonstration of in-benchmark stock selection skill
- Longer-term stability of fund managers
- Investment style persistency
- Quality risk-adjusted returns
- Longer-term outperformance versus the benchmark
Frequency of Underlying Fund Review
After constructing the initial mix of funds for a new EAM strategy, Pegassets will periodically engage in a systematic review of the strategy and all Underlying Funds. The review cycle is typically monthly for a topline review, with a more focused review on a quarterly basis.
This review includes an analysis of the performance of a strategy relative to its benchmark, peer group and the Underlying Funds, as well as consistency in the style of the strategy versus its designated style box. Further, we monitor on an ongoing basis the performance of each fund/manager’s largest overweight positions vs the benchmark. (This data is provided by Turing Technology Associates and is based on their fund replication technology, Hercules.aiTM)
A review cycle can result in a decision for no changes or might trigger removal and replacement for one or more funds. A change of more than three Underlying Funds at a time is rare. For replacement funds, they will be selected based on a similar methodology as described below.
Regarding the timing of review cycles, our experience has shown that more frequent reviews rarely prove constructive. One of the benefits of a filtering process based on longer manager tenure is that it provides proven managers an opportunity to demonstrate their stock selection skill over a reasonable period.
Further, our expectation is that the Underlying Funds will not all be outperforming at the same time. One of our core philosophies is to embed diversification of investment process, which will result in relative outperformance by fund to vary across different market cycles and conditions. This approach has been proven to Pegassets as being a key contributor to overall stability of outperformance.
Core Fund Screening Methodology
Our fund screening methodology is composed of several key steps. These include:
- Apply negative filters, designed to remove funds that would have a very low likelihood of inclusion in a Pegassets EAM strategy.
- Filter and rank funds based on the success rate of outperformance of their high conviction overweight stock picks.
- Filter and rank funds based on risk-adjusted “quality” metrics
- Filter and rank funds based on long-term annual peer group rankings to identify funds with high persistency in the top half of peer group rankings.
- Further review and filter for desired portfolio characteristics such as tenure, number of holdings and turnover.
- Review correlation of annual performance over time in order to achieve diversity, with the goal of avoiding large swings in returns. Weighing all factors, make a final selection of 12 funds.
For a more detailed description of our Fund Screening Methodology, please contact us.
We then conduct a multi-year backtest of the resulting list of fund finalists, to ensure that the funds both translate properly into an EAM environment, and that the backtested returns have the desired relative performance success criteria. Results are reviewed over various historical time periods to ensure the strategy meets targets and objectives. The review includes strategy performance relative to the benchmark, category peers and the Underlying Funds, persistency of excess return, risk measures (e.g. standard deviation, Sharpe ratio, etc.), alpha and beta levels, upside/downside capture ratios and category ranking. When the desired set of characteristics is reached, the strategy is put into production.
Targeted Objectives
Our optimal backtest results would include a rolling period success rate versus the benchmark of more than 70%, and annual excess returns over these rolling periods of between 300 to 500 basis points. (Large Blend and Large Growth have proven to be tougher benchmarks to outperform over the past decade, and thus are likely to have lower relative performance metrics.)
Contact Pegassets
- Kathleen Neumann, President: kathy.neumann@pegassets.com
- Robert Tull, Founder: robert@pegassets.com